the Creative Commons Attribution 4.0 License.
the Creative Commons Attribution 4.0 License.
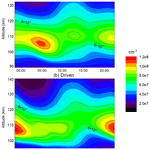
On the relative roles of dynamics and chemistry governing the abundance and diurnal variation of low-latitude thermospheric nitric oxide
David E. Siskind
McArthur Jones Jr.
Douglas P. Drob
John P. McCormack
Mark E. Hervig
Daniel R. Marsh
Martin G. Mlynczak
Scott M. Bailey
Astrid Maute
Nicholas J. Mitchell
We use data from two NASA satellites, the Thermosphere Ionosphere Energetics and Dynamics (TIMED) and the Aeronomy of Ice in the Mesosphere (AIM) satellites, in conjunction with model simulations from the thermosphere-ionosphere-mesosphere-electrodynamics general circulation model (TIME-GCM) to elucidate the key dynamical and chemical factors governing the abundance and diurnal variation of lower thermospheric nitric oxide (NO) at near-solar minimum conditions and low latitudes. This analysis was enabled by the recent orbital precession of the AIM satellite which caused the solar occultation pattern measured by the Solar Occultation for Ice Experiment (SOFIE) to migrate down to low and mid-latitudes for specific periods of time. We use a month of NO data collected in January 2017 to compare with two versions of the TIME-GCM; one is driven solely by climatological tides and analysis-derived planetary waves at the lower boundary and is free running at all other altitudes, and the other is constrained by a high-altitude analysis from the Navy Global Environmental Model (NAVGEM) up to the mesopause. We also compare SOFIE data with a NO climatology from the nitric oxide empirical model (NOEM). Both SOFIE and NOEM yield peak NO abundances of around 4×107 cm−3; however, the SOFIE profile peaks about 6–8 km lower than NOEM. We show that this difference is likely a local time effect, with SOFIE being a dawn measurement and NOEM representing late morning and/or near noon. The constrained version of TIME-GCM exhibits a low-altitude dawn peak, while the model that is forced solely at the lower boundary and free running above does not. We attribute this difference to a phase change in the semi-diurnal tide in the NAVGEM-constrained model, causing the descent of high NO mixing ratio air near dawn. This phase difference between the two models arises due to differences in the mesospheric zonal mean zonal winds. Regarding the absolute NO abundance, all versions of the TIME-GCM overestimate this. Tuning the model to yield calculated atomic oxygen in agreement with TIMED data helps but is insufficient. Furthermore, the TIME-GCM underestimates the electron density (Ne) as compared with the International Reference Ionosphere (IRI) empirical model. This suggests a potential conflict with the requirements of NO modeling and Ne modeling, since one solution typically used to increase model Ne is to increase the solar soft X-ray flux, which would, in this case, worsen the NO model–data discrepancy.
- Article
(2116 KB) - Full-text XML
- BibTeX
- EndNote
Nitric oxide (NO) has long been recognized as one of the most important trace constituents in the middle and upper atmosphere. This is due to its role in cooling the thermosphere through mid-infrared emission (Kockarts, 1980; Mlynzcak et al., 2003; Knipp et al., 2017), as a source for NO+ ions in the lower ionosphere (Solomon, 2006) and more generally as an indicator of energy input into the atmosphere (Siskind et al., 1989b; Barth et al., 1999; Mlynczak et al., 2018a). Motivated by the development of whole atmosphere models and the availability of new datasets, there has been much recent work on the properties and role of NO at high latitudes. Here NO can serve both as measure of energetic particle precipitation (EPP) into the atmosphere (Hendrickx et al., 2015, 2018; Smith-Johnson et al., 2017) and as a tracer for descent in the winter polar vortex (Bailey et al., 2014; Randall et al., 2015; Siskind et al., 2015; Newnham et al., 2018), ultimately coupling with the chemistry of the mesosphere and stratosphere (Funke et al., 2017). There has been less recent work on low-latitude nitric oxide, although it has recently been shown that equatorial NO can be used as a diagnostic of non-migrating tides (Oberheide and Forbes, 2008; Oberheide et al., 2013). The primary emphasis of this work will be on equatorial NO, its absolute abundance and its diurnal variability.
Most of the extant thermospheric NO measurements are limited to specific local times. This results either from satellites in sun-synchronous orbits or from satellites which might be in varying local time orbits but use a technique such as solar occultation that is inherently limited to a single local time. Examples of the first case include the Student Nitric Oxide Explorer (SNOE; Barth et al., 2003), the Michelson Interferometer for Passive Atmospheric Sounding (MIPAS) dataset on the European research satellite Envisat (Bermejo-Pantaleón et al., 2011; Bender et al., 2015) and the submillimeter radiometer (SMR) on the Swedish Odin satellite (Sheese et al., 2013; Kiviranta et al., 2018). Examples of the second case include the Solar Occultation for Ice Experiment (SOFIE; Gómez-Ramírez et al., 2013) on the Aeronomy of Ice in the Mesosphere (AIM; Russell III et al., 2009), the ACE Fourier Transform Spectrometer (ACE-FTS; Bernath et al., 2005; Bender et al., 2015) and the Halogen Occultation Experiment (HALOE) data on the NASA Upper Atmosphere Research Satellite (UARS; Russell III et al., 1993; Siskind et al., 1998). We thus deduce that, unfortunately, there is no satellite data which can directly resolve the diurnal variation of thermospheric nitric oxide. Therefore any exploration of this variation must necessarily be indirect. Here, with the assistance of a thermospheric general circulation model, we present such an indirect approach. Specifically, we compare SOFIE data with data from SNOE, as encapsulated in the nitric oxide empirical model (NOEM; Marsh et al., 2004). As noted above SOFIE measures at either sunrise or sunset; SNOE data were acquired at about 11:00 LT. To compare the two we will use diurnally resolved model results from the thermosphere–ionosphere–mesosphere-electrodynamics general circulation model (TIME-GCM) as recently described by Jones Jr. et al. (2018). One additional, important aspect to our model data comparisons are that they are multi-constituent. To understand how the diurnal variation of nitric oxide might be sensitive to migrating tidal amplitudes, we will compare our model results with observations of upper mesospheric zonal winds (deWit et al., 2013). Furthermore, since the abundance of NO is known to be sensitive to atomic oxygen (Siskind et al., 1989a), we will compare TIME-GCM output to Sounding of the Atmosphere using Broadband Emission Radiometry (SABER) atomic oxygen (O) data. Finally, since the ionization that ultimately leads to nitric oxide also produces the E-region ionosphere (Solomon et al., 2001; Sojka et al., 2013), we will compare our model output to the International Reference Ionosphere (IRI; Bilitza, 2015). In this manner, we will significantly reduce the number of free parameters to guide future model development.
For most of the AIM mission, the SOFIE occultations have been confined to high latitudes, consistent with the focus of the AIM on polar mesospheric clouds. However, recently, due to the precession of the AIM orbit, this occultation pattern has occasionally migrated to lower latitudes. Of specific interest for this work is the period from December 2016–January 2017, where SOFIE occultations made at local sunrise (05:00–06:00 LT) were confined to near equatorial latitudes (3–4∘ S). To quantitatively analyze this period, we are forced to make some key assumptions about the causes of NO variability in the tropics. This is because the TIME-GCM model we use is constrained by meteorological data from January 2010, not January 2017. However, at tropical latitudes, when averaged over a month, it is reasonable to assume that the average NO will be governed by solar and geophysical forcing. Figure 1 shows the variation of the solar and geomagnetic activity indices (obtained via anonymous FTP from the US National Geophysical Data Center at ftp://ftp.ngdc.noaa.gov/, last access: 21 January 2019) for both months of January. It shows that for both months, solar activity was, on average, low, although it was slightly higher than the absolute solar minimum (cf. https://www.swpc.noaa.gov/products/solar-cycle-progression, last access: 21 January 2019). The average F107 solar flux index was 78 for January 2010 and 75 for January 2017. Likewise, geomagnetic activity was equally low: the averaged geomagnetic Ap index was 9 for 2017 and 3 for 2010. These differences are very small, thus we argue that while the day-to-day NO might vary due to meteorological forcing from below, when averaged over a month, there should be little difference in the NO profiles for January 2010 and 2017. A possible limitation of this assumption will be addressed in the Discussion section below.
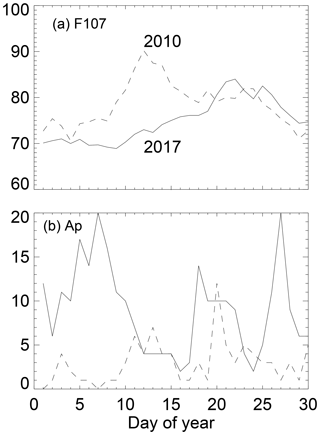
Figure 1(a) Daily variation of the solar F107 index for January 2017 (solid) and 2010 (dashed). (b) Daily Ap for the same period.
Figure 2 shows the monthly averaged, zonally averaged SOFIE profile compared with the NOEM results computed for 2010 and 2017. First, the NOEM results for the 2 years are almost identical, and this supports our arguments above about the acceptability of comparing the 2 years. Second, both SOFIE and NOEM give peak NO densities of about 4.5×107 cm−3; however, the peak in the SOFIE NO is displaced downward by about 8 km from NOEM (note: the NOEM profile peaks at 110 km, and an examination of individual SNOE profiles, from which NOEM is derived, often show the peak altitude closer to 108 km – not shown). Regardless, this difference between SOFIE and SNOE or NOEM of either 6 or 8 km is significantly greater than the 2–3 km altitude resolution of either instrument). As we will discuss below, this altitude difference likely reflects the local time difference between the SOFIE data (05:00–06:00 LT) and SNOE, as encapsulated by NOEM (near 11:00 LT). Above the peak, SOFIE NO is lower than NOEM, but at the higher altitudes, above 120 km, SOFIE appears to approach the NOEM values. The figure shows the 1σ variability of the SOFIE data about the monthly mean; we feel this is the best measure of uncertainty in the SOFIE data. Individual errors in the SOFIE profiles are no more than 25 % (cf. Table 1 of Goméz-Ramírez et al., 2013). Since the SOFIE data in the figure represent an average of over 400 profiles (15 per day for about 30 days), any random error will be reduced to insignificant values, leaving geophysical variability as the only quantifiable uncertainty in the data. These three facets of the data, the peak magnitude, the altitude of the peak and the behavior at the higher altitudes, will be the subject of the model–data comparisons below.
3.1 Background on the TIME-GCM
The NCAR TIME-GCM is one of several NCAR global general circulation models of the middle and upper atmosphere. It self-consistently (Roble and Ridley, 1994) solves the momentum, energy, continuity and electrodynamic equations from the first principles for the global circulation, temperature, composition, and electrodynamics of the mesosphere, thermosphere and ionosphere on a regular grid in spherical coordinates in longitude and latitude and log pressure in the vertical grid, assuming hydrostatic balance. The resolution of the TIME-GCM is 2.5∘ × 2.5∘ (longitude × latitude) and four grid points per vertical scale height, extending from 12 to hPa (or 30 to 450–600 km, depending upon solar activity). Geomagnetic forcing is parameterized using the 3 h Kp index, consistent with the 2010 Ap values shown in Fig. 1. Further details are given by Jones Jr. et al. (2018).
3.2 Overview of approach
We have adapted the TIME-GCM so that we can compare two approaches towards modeling the mesosphere and lower thermosphere (MLT). The standard version of the TIME-GCM uses a combination of the Global Scale Wave Model (GSWM; Zhang et al., 2010a, b) as a bottom boundary (approximately 30 km) for the diurnal and semi-diurnal tidal forcing and the daily averaged European Centre for Medium-Range Weather Forecasts (ECMWF) analysis for the planetary wave distribution. We will call this the “driven” model herein. We will compare this driven model with a version of the model where it is nudged to the winds and temperatures from the high-altitude version of NAVGEM (Navy Global Environmental Model, described in McCormack et al., 2017). The nudging technique is described more fully by Jones Jr. et al. (2018). As they discuss, at the lower boundary of the TIME-GCM, the nominal GSWM and ECMWF fields are replaced with the NAVGEM analyses using a normalized weighting factor of unity. This weighting factor decreases with increasing altitude and becomes zero at the top of the NAVGEM analysis, at approximately 95 km. Above this altitude, the TIME-GCM is free running.
In addition, to more fully explore the possible roles of dynamics and chemistry on the NO, we performed two additional simulations with the NAVGEM-nudged model where we perturbed some key input parameters. One variation was to reduce the vertical eddy diffusion (Kzz) coefficient by a factor of 10; the other was to increase the quenching of metastable atomic nitrogen N(2D) by a factor of 2. The rationale behind both these changes was to reduce the calculated NO abundance, which, as we show below, is too large in the baseline cases. This can be understood by considering the chemistry of N(2D). In the MLT, N(2D) can either react with molecular oxygen to produce NO according to
or it can be quenched by atomic oxygen according to
Reaction (R2) is then followed by
which is the ultimate sink of nitric oxide. Thus to reduce the calculated NO, we aim to increase the rate of Reaction (R2) at the expense of Reaction (R1). As we show below, we did this by either increasing the calculated atomic oxygen or by increasing the rate coefficient governing R2. The standard TIME-GCM model uses a value for Reaction (R2) of cm3 s−1 from Fell et al. (1990). As discussed by Yonker (2013), Herron (1999) inferred a temperature dependence of this rate that appears to neglect the Fell et al. (1990) reference. Instead, relying upon older studies, Herron (1999) recommends a room temperature value which is about double the room temperature measurement of Fell et al. (1990). Thus in doubling the rate coefficient R2 we are essentially, as an academic exercise, using Herron's somewhat arbitrary room temperature value in lieu of Fell et al.'s (1990) measurement.
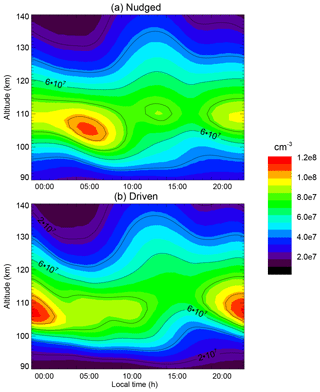
Figure 3Diurnal variation of calculated nitric oxide at 4∘ S. (a) The model nudged by NAVGEM, and (b) the model driven at the bottom boundary by the GSWM model and the MERRA analysis. The contour interval is 2×107 cm−3.
Figure 3 compares the diurnal NO variation between the driven and nudged models. It shows that both models have peak NO densities in the lower thermosphere of just over 108 cm−3. The nudged model peak occurs at sunrise, while the driven model's peak occurs at midnight. Two points are evident from these simulations. First, it is apparent that the overall magnitude of the peak NO density is much greater than the observations. Second, the nudged model displays a peak at sunrise (i.e., the local time of the SOFIE observation), which is 4–5 km lower in altitude than it is at other times of the day. After about 08:00 LT and into the early afternoon, the altitude of the peak rises. This seems qualitatively consistent with the difference between SOFIE and NOEM. By contrast, for the driven model, the peak NO is at midnight, and there is no change in altitude between sunrise and early afternoon. To attempt to see how diurnal variation in the two models might compare with the SOFIE∕NOEM difference, we took the ratio of the equatorial sunrise NO in the models (average of 05:00–06:00 LT) with the 11:00 LT profiles (SNOE local time) and plot them with the ratio of SOFIE∕NOEM. This is shown in Fig. 4.
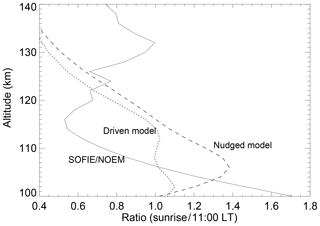
Figure 4Ratio of nitric oxide from sunrise to late morning at 4∘ S. For the two models, this was done by taking the ratio of the nitric oxide averaged from 05:00–06:00 LT (to approximate the local time of the SOFIE observations) to that at 11:00 LT (roughly the local time of the SNOE observations). Also shown as the solid line is the ratio of the SOFIE data to the NOEM 2017 model shown in Fig. 2.
The figure shows that the SOFIE∕NOEM ratio is greatest at 100 km (about a factor of 1.7) and decreases monotonically up to about 115 km. Neither model exactly reproduces this behavior, but the slope in the nudged model between 115 and 105 km comes much closer. Like the data, the nudged model decreases with increasing altitude between 105 and 115 km, whereas the driven model ratio is nearly constant in this altitude range. The absolute value of the sunrise ∕ 11:00 LT ratio in the nudged model is also in better agreement than the driven model; it shows a peak at about 105 km of about 1.4 and decreases above this level. This is consistent with the change in peak altitude seen in Fig. 3. The ratio in the driven model barely exceeds 1.0, consistent with the lack of a change in the peak altitude between 05:00 and 11:00 LT.
Also of interest is that neither model shows the sharp turnaround in the sunrise ∕ 11:00 LT ratio seen in the SOFIE∕NOEM comparison above 115–120 km. This turnaround reflects the fact that the SOFIE values are approaching NOEM at these altitudes, while both models suggest that the dawn values should be much lower than those nearer noon. Since the NO variation in the model above 120 km is driven solely by the nighttime recombination of NO with N(4S) according to Reaction (R3) above, it is difficult to imagine a scenario whereby the dawn NO should be larger than midday. This change in slope could be driven by a lower signal-to-noise ratio in the retrieval at the higher altitudes; however, the SOFIE curve in Fig. 2 represents an average of about 400 profiles, which should reduce the noise effects. It could also result from an bias that is still unidentified at these altitudes. More work will be needed to clarify this.
3.3 Absolute magnitude
The analysis above suggests that the difference between the nudged and driven model may shed light on the differences between SOFIE and SNOE (as reflected in NOEM) and thus on the NO diurnal cycle. However, we must first address the question as to why the observed NO magnitude is so much smaller than in the models. We first explore the possibility that the model atomic oxygen is discrepant with observations.
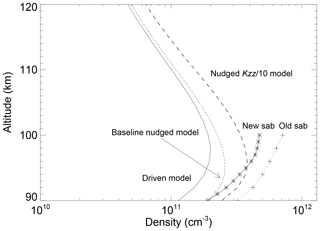
Figure 5Monthly averaged atomic oxygen profiles from three simulations with the TIME-GCM and two versions of the SABER database. The curve labeled old sab is the Version 2 data of Mlynczak et al. (2013); the curve labeled new sab is the reprocessed data described by Mlynczak et al. (2018b).
Figure 5 shows the calculated atomic oxygen profiles for the Equator from the driven and nudged models. Also shown are two profiles from the SABER instrument. The curve labeled “old sab” is the standard Version 2 product described by Mlynczak et al. (2013). The curve labeled “new sab” represents a reprocessing of that data using new kinetics, as discussed by Mlynczak et al. (2018b). Although the new SABER is lower than the old, both profiles still exceed the driven and nudged models at all altitudes above 90 km and at 100 km, and near the peak of the NO, the difference is almost a factor of 3. This means that the driven and nudged models will necessarily underestimate the rate of R2. Since R2 competes with R1, underestimating R2 means an overestimate of R1 and will thus lead to overestimating the production of NO. Therefore increasing the model O should reduce the model NO. To increase the model atomic oxygen, we ran the nudged model with Kzz (originally set to the nominal TIME-GCM values used by Jones Jr. et al., 2017) divided by a factor of 10. As discussed by Jones Jr. et al. (2017), Siskind et al. (2014) and earlier, by Forbes et al. (1993), reducing downward vertical transport, in this case by reducing the eddy diffusion, will reduce the recombination of atomic oxygen in the mesosphere and thus lead to increased O in the lower thermosphere. Siskind et al. (2014) further argue that reducing Kzz can be acceptable for models which incorporate a more realistic spectrum of variable dynamical forcing from the lower atmosphere, as in the nudged case here. Figure 5 shows that the atomic oxygen for the nudged Kzz∕10 case is about a factor of 2 greater than the other models and is within 30 % of the new SABER values.
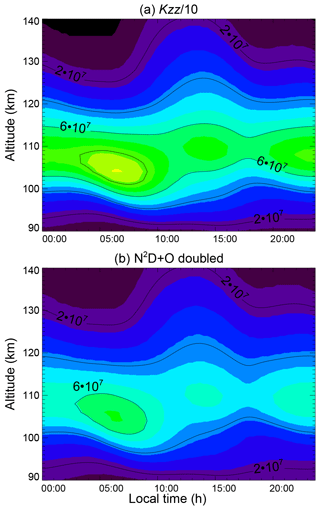
Figure 6Diurnal variation of calculated nitric oxide at 4∘ S, both with the nudged model, but with the additional indicated changes to the TIME-GCM. The color scheme is the same as in Fig. 3
Figure 6 shows the diurnal variation of the calculated NO from the Kzz∕10 model and also from the model where the reaction rate coefficient for Reaction (R2) above was arbitrarily increased by a factor of 2. Both models show significantly less NO than the baseline nudged model shown in Fig. 3. Doubling the rate of R2 was more effective in getting the NO magnitude down to the 4–5 ×107 cm−3 range seen in SOFIE and NOEM. However, increasing atomic oxygen to agree better with SABER still did reduce the NO by about 50 %. Importantly, both simulations still show the peak NO at sunrise, with a lifting of the layer peak in late morning. Thus this diurnal variation is seen to be somewhat robust against changes in the NO chemistry. As we will discuss in the next section, the dawn peak is related to tidal oscillations.
3.4 Diurnal variation
The NO maxima in the TIME-GCM, whether at sunrise in the NAVGEM-nudged model or at midnight in the driven model, are clearly associated with descent. This can be seen by looking at Fig. 7, which shows the diurnal variation of three indicators of vertical motion in the lower thermosphere: the temperature, the O∕O2 ratio and the nitric oxide mixing ratio. All three of these quantities increase with altitude, thus a local increase at a single pressure level reflects vertical transport downwards from higher altitudes. The assumption that NO can be treated as a passive tracer to study tidal variability was first discussed by Marsh and Russell III (2000) and most recently by Oberheide et al. (2013); it is particularly valid for the nighttime and dawn conditions discussed here where chemical damping is at a minimum. Figure 7 clearly shows that all three of these quantities show a peak near 06:00–07:00 LT in the nudged model. Furthermore, by late morning these quantities decrease, consistent with upward motion. By contrast, in the driven model, they show a peak at midnight and minima at dawn. Comparing the vertical transport implied by Fig. 7 with the calculated NO densities in Fig. 3, it is clear that the maximum in downward transport corresponds to the NO maxima shown in Fig. 3. We can then further interpret the low-altitude NO peak seen in SOFIE, relative to NOEM, as reflecting the diurnal variation of NO such that descent occurs in the early morning and this reverses in the late morning, when SNOE measured NO.
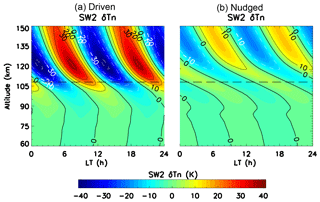
Figure 8Variation of SW2 temperature tide vs. altitude for (a) the GSWM-driven model and (b) the NAVGEM-nudged model.
To understand the differences in the vertical transport in both models, we first note that a dominant mode of variability is a semi-diurnal oscillation. This is most evident in the driven model, but it is apparent (and confirmed by spectral analysis, not shown) in the nudged model. Thus we investigate the differences in the migrating semi-diurnal tide (SW2) in the two models. Figure 8 depicts the local time variation of SW2 temperature versus altitude calculated via a 2-D Fourier decomposition of the TIME-GCM temperature fields. It is clear that quite different solutions are reached between the two TIME-GCM models. In the driven case, the amplitude in the lower thermosphere (we use 108 km as a reference altitude) is quite large, at about 25 K. This is well in excess of observations presented by Akmaev et al. (2008) and theoretical calculations presented earlier by Forbes and Vial (1991), whereas in the nudged case it is in good agreement with those references. Also apparent is a distinct difference in the tilt of the lines of constant phase in the tide in the nudged case compared with the driven case. This is consistent with a change in the vertical wavelength such that it remains quite large in the driven case (>50 km) but becomes smaller in the nudged case. As we show below, these amplitude differences are associated with a significant phase difference between the calculated SW2 in the driven and nudged models. We attribute these phase differences to the underlying zonal wind.
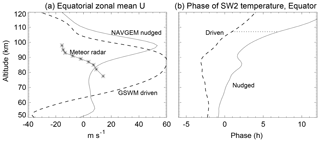
Figure 9(a) Vertical profiles of monthly averaged, zonally averaged TIME-GCM zonal winds for the GSWM-driven model (dashed line) and the NAVGEM-nudged model (solid line). Also shown for comparison are monthly averaged meteor radar winds for January 2010 taken from Ascension Island (8∘ S, 14.4∘ W). (b) Associated phase of the SW2 tide for the two TIME-GCM simulations. The thin dotted line is simply a reference fiducial to show the phase difference between the two simulations at 108 km.
Figure 9 shows the qualitative link between the vertical profile of the zonal winds and the phase of SW2 from the mesosphere to the lower thermosphere. The left-hand panel shows the monthly averaged, zonally averaged equatorial zonal wind profile from the driven and nudged models and compares them with radar winds taken from Ascension Island (latitude is 8∘ S; see McCormack et al., 2017, for further discussion of the wind data). Neither model exactly reproduces the data, but the nudged model does better in that it somewhat suppresses the very large and vertically broad layer of strong eastward wind seen in the driven case. The differences in the zonal winds are consistent with those discussed by Jones Jr. et al. (2018) and are most likely attributed to a large eastward momentum source produced by the TIME-GCM's gravity wave drag parameterization. The right-hand panel of Fig. 9 shows how the phase of the SW2 becomes increasingly different between the two models in the altitude region where the winds are different. At 108 km, as illustrated by the short dashed line, this difference is about 6 h, i.e., the SW2 in the nudged model has become almost exactly out of phase with that in the driven model. This is completely consistent with the diurnal variation of vertical transport shown in Fig. 7, where the descent in the driven model peaked near midnight and that for the nudged case peaked 6 h later, around sunrise.
Based upon theoretical work by Forbes (2000) and Forbes and Vincent (1989) on the effects of mean winds on atmospheric waves, we understand that both the phase and amplitude of the SW2 tide will be sensitive to the background zonal wind field through which it propagates. Specifically, as discussed by Forbes (2000) a wave propagating in the opposite direction of the mean wind, as is the case here, will see an increased vertical wavelength and decreased damping. Thus the greater eastward zonal winds seen in the driven model will correspond to a longer wavelength in the SW2 tide and to a greater amplitude. Since the winds in the driven case are much greater than observed, it stands to reason that the amplitude of the tide is much greater than observed.
Our model–data comparison has implications for our understanding of both the dynamics and the chemistry of the low-latitude MLT. First, our work illustrates the need for an accurate simulation of the mesospheric zonal winds in calculating the diurnal variation of NO. It is probably not surprising that the simulation of the mesospheric zonal winds affects the propagation of tides up from the middle atmosphere. Jones Jr. et al. (2018) cover aspects of this topic using the TIME-GCM with different nudging scenarios, and the theoretical studies we cited above have presented analytic explanations for several decades. However, in illustrating how variations in the SW2 tide can affect the variation of nitric oxide, we have presented a new mechanism for whole atmosphere coupling from the middle atmosphere to the thermosphere.
We should note that although we have discussed the semi-diurnal tide, we only have emphasized its effect in the early morning. For a tide which peaks twice per day (like SW2), in principle, one might expect effects 12 h later, in the late afternoon, which, however, is not as noticeable (compare the differences between nudged and driven models in Fig. 7 at 18:00 vs. 06:00 LT). We suggest that the major reason why the sunrise difference is more apparent than the sunset difference lies in the relative roles of dynamics versus chemistry. In the pre-dawn hours, NO is essentially a passive tracer. Thus changes in vertical motion translate directly into changes in NO abundance. However in the afternoon, under the influence of extreme ultraviolet (EUV) and X-ray solar illumination, NO is under more chemical control. Differences in vertical motion should have less of an impact. We also compared the phase variations of the migrating diurnal and terdiurnal tidal modes (DW1 and TW3, respectively) between the driven and nudged models, and they showed little difference (not shown); in any event, their amplitudes were smaller than the SW2 which we emphasize here. Thus all our models show a distinct difference, preferentially in the sunrise abundances, which we link to a phase change in the SW2. One final consideration here is that although we argued that the drivers of NO variability between 2010 and 2017 were essentially identical, the meteorological forcing might not have been. Thus there was a sudden stratospheric warming (SSW) on 27 January 2010, and similar dynamics were absent in 2017. Pedatella and Liu (2013) showed that SSW events can induce phase changes in the SW2 tide. However, these changes were limited to generally less than 2 h in phase, which is less than we show here. Furthermore, the 2010 SSW occurs at the tail end of our averaging period, thus it is likely that for most of the month, the dynamical forcing was not that different between 2010 and 2017. All this is important because satellites such as ODIN are in sun-synchronous orbits and acquire both sunrise and sunset data. The recent ODIN-based model of Kiviranta et al. (2018) did not distinguish between sunrise and sunset data; our results suggest it would be useful to do so.
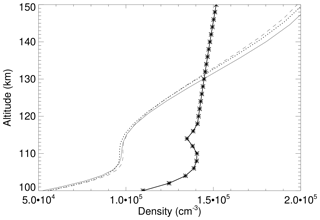
Figure 10Altitude variation of electron density from three TIME-GCM simulations as compared with IRI, all for noon at the Equator. The thick solid line with stars is IRI, the thin solid line is the standard nudged model, the short dotted line is the nudged with Kzz∕10 model and the dashed line is the nudged model with the reaction rate coefficient for N(2D)+O doubled.
More problematical than the diurnal variability might be the question of the absolute abundance of the calculated nitric oxide which is significantly overestimated by the model. Interestingly, Hendrickx et al. (2018) encountered the same problem with the Whole Atmosphere Community Climate Model (WACCM) compared with SOFIE in the auroral zone. Hendrickx et al. (2018) also mentioned the importance of capturing the correct compositional abundances of the background atmosphere, specifically the atomic oxygen. Here we show that tuning the model to better match SABER, while certainly necessary, appears insufficient. Even with O being in reasonable agreement with SABER, the model still significantly overestimated the NO. Conventionally, in this situation, the other unknown that NO modelers focus on is the solar flux (Siskind et al., 1990, 1995), specifically the soft X-rays that ionize and dissociate N2 in the lower thermosphere. However, in this case, the presumed remedy to reduce the model NO would be to reduce the soft X-ray flux, and this would likely make the calculation of the E-region electron densities worse. The reason is illustrated in Fig. 10, which shows three TIME-GCM model simulations compared with IRI, all for noon at the Equator. Note how below 125 km, where soft X-ray ionization becomes important, the models all underestimate IRI. This is a robust comparison because a new empirical model, the 2018 Faraday-IRI model of Friedrich et al. (2018), shows E-region electron densities using a different dataset than used by IRI and gets similar answers, i.e., for high-sun conditions at 100 km, the electron density equals or exceeds 105 cm−3. This model underestimate of the E-region electron density has been recognized before (Maute, 2017, and references therein) and is the reason that those authors increased the soft X-ray flux. Pavlov and Pavlova (2015) had the same problem and made the same change to the reference soft X-ray spectrum, i.e., they increased it. Thus it appears that the requirements of the nitric oxide simulation and the E-region electron density simulation are in conflict.
To resolve the model overestimate of nitric oxide without worsening the model underestimate of the E region Ne, we can suggest two possibilities. The first is that aspects of the nitric oxide kinetics should be reevaluated. We have shown that increasing the N(2D)+O rate can bring the model NO into agreement with SOFIE and NOEM. As we discussed above, concerning Reaction (R2), the evidence for this particular rate coefficient being underestimated by a factor of 2 is mixed at best; our adoption of this faster rate could be best considered as a proxy for other, still unidentified changes to the odd nitrogen kinetic scheme. A second possibility is perhaps more speculative but is intriguing in that it might solve two problems at once. This would be a scenario whereby the E-region production of is increased. The could then serve as a partial sink for nitric oxide via
The sink is described as only “partial”, because the recombination of NO+ favors N(2D) so that much of the NO will be reformed (Yonker, 2013). However, it could go in the right direction. Conventionally, much of the production in the E region is from the strong solar Lyman β line. As with the rest of our solar spectrum, we use Solomon and Qian (2005), which for the band that includes Lyman β (987.7–102.7 nm), gives a near-solar minimum flux of about 4.5×109 cm−2 s−1. This is unlikely to be an underestimate when compared with other datasets such as that discussed by Warren (2005) and Warren et al. (1998), so we are constrained from making a drastic change (such as a doubling) to the flux at these wavelengths. An alternative idea was proposed by Meier et al. (2007), who suggested that the use of averaged cross sections could have the effect of underestimating the penetration of some of the solar EUV spectrum down to 110 km. They show that the O+ ionization in the E region could be significantly increased with higher resolution. Interestingly, the wavelengths they discuss are those which ionize O2 but not N2. Thus although Meier et al. (2007) did not show O2 ionization, it seems plausible that their high-resolution cross sections would lead to more O2 ionization in the E region. This should be given some consideration for future work.
Taken together, the indirect comparison of SOFIE with NOEM shows that we can reasonably define a baseline minimum value of the NO peak density, approximately equal to 4×107 cm−3, representing solar minimum conditions at the Equator for low levels of geomagnetic activity. Furthermore we have provided some insight into the possible reason for the approximate 8 km altitude offset between the peak NO seen by SOFIE and NOEM. This difference appears to be dynamically driven, specifically due to the 6 h phase change of the migrating semi-diurnal tide as it propagates up from the stratosphere to the lower thermosphere. While the TIME-GCM as nudged by NAVGEM does not give an exact replication of the sunrise ∕ 11:00 LT NO ratio indicated by the SOFIE–NOEM comparison, it does support the existence of a low-altitude NO peak at dawn. Our results further suggest that a more accurate simulation of the zonal wind would likely give a better simulation of the sunrise ∕ 11:00 LT NO ratio.
Our calculation of the absolute abundance of nitric oxide significantly exceeds both the SOFIE and NOEM values. What is new to our approach here is that we simultaneously compare our calculation to the SABER atomic oxygen data as well as to empirical models of the E-region ionosphere. This kind of comparison significantly constrains the number of free parameters; in the case where we tune the model to improve agreement with SABER, we also improve the agreement with SOFIE and NOEM. However, lowering the soft X-ray flux to further reduce the NO would likely worsen the model underestimate of Ne. We thus suggested an alternative, admittedly more speculative remedy involving the use of higher-resolution cross sections to increase E-region ionization. Regardless of the specific remedy to the discrepancy, our study points to the value of a multi-constituent approach (i.e., O, NO and Ne) towards validating models such as the TIME-GCM and demonstrates the utility of nitric oxide as a useful diagnostic of chemical and dynamical processes at the base of the thermosphere.
The SOFIE NO data can be obtained by FTP from ftp://ftp.gats-inc.com/sofie (last access: 21 January 2019, GATS Inc., 2019a) and the SABER O data can be obtained at http://saber.gats-inc.com/data.php (last access: 21 January 2019, GATS Inc., 2019b). NOEM is distributed as part of the NCAR∕GLOW model (Stan Solomon, PI) and can be downloaded from https://github.com/NCAR/GLOW (last access: 21 January 2019, Solomon, 2017). Daily NCAR TIME-GCM outputs in netCDF format from this study are archived on the Department of Defense (DoD) HPCMP long-term storage system. The NAVGEM inputs used to constrain the TIME-GCM simulations presented here have been placed on the repository described by Jones Jr. et al. (2018; i.e., https://map.nrl.navy.mil/map/pub/nrl/james2018, last access: 21 January 2019).
DES did the model–data comparisons and wrote the main body of text. MJ Jr. provided the model results, did the SW2 analysis and helped edit the text. DPD assisted in configuring the TIME-GCM simulations and advised on the TIME-CM nudging scheme. JPM advised on the NAVGEM model results, their range of validity and comparison with radar winds. MEH advised on the SOFIE data and their range of validity and helped edit the text. DRM assisted with the NOEM code and advised on the model–data comparison. MGM advised on the range of validity of the new SABER O data and helped edit the text. SMB advised on the comparison of NOEM and SOFIE and helped edit the text. AM advised on the use of the TIME-GCM to calculate electron density. NJM advised on the use of the Ascension Island wind data.
The authors declare that they have no conflict of interest.
We acknowledge support from the NASA AIM Small Explorer program (through the
Interagency Purchase Request S50029G to NRL), the NASA/TIMED SABER project
(through Interagency Purchase Request NNG17PX04I to NRL) and the Office of
Naval Research BSION program, award number
N0001417WX00579. Additionally, Douglas P. Drob acknowledges support from the
NASA Heliophysics Supporting Research (HSR) program through interagency
agreement NNH17AE69I to NRL. This work was performed while McArthur Jones Jr.
held an NRL Karle Fellowship. John P. McCormack
acknowledges support from NASA grant NNH13AV95I. Astrid Maute is supported by
NASA grants X13AF77G and NNX16AG64G. Computational resources for this work
were provided by the U.S. Department of Defense (Dod) High Performance
Computing Modernization Program (HPCMP). We thank Stan Solomon of the High
Altitude Observatory (HAO) for helpful discussion concerning nitric oxide
chemistry and the internal reviewer at HAO for useful comments. NCAR is
supported by the National Science Foundation.
Edited by: Petr Pisoft
Reviewed by: Koen Hendrickx and two
anonymous referees
Akmaev, R. A., Fuller-Rowell, T. J., Wu, F., Forbes, J. M., Zhang, X., Anghel, A. F., Iredell, M. D., Moorthi, S., and Juang, H.-M.: Tidal variability in the lower thermosphere: Comparison of Whole Atmosphere Model (WAM) simulations with observations from TIMED, Geophys. Res. Lett., 35, L03810, https://doi.org/10.1029/2007GL032584, 2008.
Bailey, S. M., Thurairajah, B., Randall, C. E., Holt, L., Siskind, D. E., Harvey, V. L., Venkataramani, K., Hervig, M. E., Rong, P. P., and Russell III, J. M.: A multi tracer analysis of thermosphere to stratosphere descent triggered by the 2013 stratospheric sudden warming, Geophys. Res. Lett., 41, 5216–5222, https://doi.org/10.1002/2014GL059860, 2014.
Barth, C. A., Bailey, S. M., and Solomon, S. C.: Solar-terrestrial coupling: Solar soft X-rays and thermospheric nitric oxide, Geophys. Res. Lett., 26, 1251–1254, 1999.
Barth, C. A., Mankoff, K. D., Bailey, S. M., and Solomon, S. C.: Global observations of nitric oxide in the thermosphere, J. Geophys. Res., 108, 1027, https://doi.org/10.1029/2002JA009458, 2003.
Bender, S., Sinnhuber, M., von Clarmann, T., Stiller, G., Funke, B., López-Puertas, M., Urban, J., Pérot, K., Walker, K. A., and Burrows, J. P.: Comparison of nitric oxide measurements in the mesosphere and lower thermosphere from ACE-FTS, MIPAS, SCIAMACHY, and SMR, Atmos. Meas. Tech., 8, 4171–4195, https://doi.org/10.5194/amt-8-4171-2015, 2015.
Bernath, P. F, McElroy, C. T., Abrams, M. C., Boone, C. D., Butler, M., Camy-Peyret, C., Carleer, M., Clerbaux, C., Coheur, P.-F., Colin,R., DeCola, P., DeMaziere, M., Drummond, J. R., Dufour, D., Eveans, W. F. J., Fast, H., Fussen, D., Gilbert, K., Jennings, D. E., Llewellyn, E. J., Lowe, R. P., Mahieu, E., McConnell., J. C., McHugh, M., McLeod, S. D., Michaud, R., Midwinter, C., Nassar, R., Nichitiu, F., Nowlan, C., Rinsland, C. P., Rochon, Y. J., Rowlands, N., Semeniuk, K., Simon, P., Skelton, R., Sloan, J. J., Souch, M.-A., Strong, K., Tremblay, P., Turnbull, D., Walker, K. A., Walkty, I., Wardle, D. A., Wehrle, V., Zander, R., and Zou, J.: Atmospheric chemistry experiment (ACE): mission overview, Geophys. Res. Lett., 32, L15S01, https://doi.org/10.1029/2005GL022386, 2005.
Bermejo-Pantaleón, D., Funke B., López-Puertas, M., Garcia-Comas., M., Stiller, G. P., von Clarmann, T., Linden, A., Grabowski, U., Höpfner, M., Kiefer, M., Glatthor, N., Kellmann, S., and Lu, G.: Global observations of thermospheric temperature and nitric oxide from MIPAS spectra at 5.3 µm, J. Geophys. Res., 116, A10313, https://doi.org/10.1029/2011JA016752, 2011.
Bilitza, D.: The international reference ionosphere-status 2013, Adv. Space Res., 55, 1914–1927, 2015.
de Wit, R. J, Hibbins, R. E., Espy, P. J., and Mitchell, N. J.: Interannual variability of mesopause winds over Ascension Island: Coupling to the stratospheric QBO, J. Geophys. Res., 118, 12052–12060, https://doi.org/10.1002/2013JD020203, 2013.
Fell., C., Steinfeld, J. I., and Miller, S., Quenching of N(2D) by O(3P), J. Chem. Phys., 92, 4768–4777, 1990.
Forbes, J. M.: Wave coupling between the lower and upper atmosphere: case study of an ultra fast Kelvin wave, J. Atm. Sol-Terr. Phy., 62, 1603–1621, 2000.
Forbes, J. M. and Vial, F.: Semi diurnal tidal climatology of the E region, J. Geophys. Res., 96, 1147–1157, 1991.
Forbes, J. M. and Vincent, R. A.: Effects of mean winds and dissipation on the diurnal propagating tide: An analytic approach, Planet. Space Sci., 37, 197–209, 1989.
Forbes, J. M., Roble, R. G., and Fesen, C. G.: Acceleration, heating and compositional mixing of the thermosphere due to upward propagating tides, J. Geophys. Res., 98, 311–321, 1993.
Friedrich, M., Pock, C., and Torkar, K.: FIRI-18 An updated empirical model of the lower ionosphere, J. Geophys. Res., 123, 6737–6751, https://doi.org/10.1029/2018JA025437, 2018.
Funke, B., Ball, W., Bender, S., Gardini, A., Harvey, V. L., Lambert, A., López-Puertas, M., Marsh, D. R., Meraner, K., Nieder, H., Päivärinta, S.-M., Pérot, K., Randall, C. E., Reddmann, T., Rozanov, E., Schmidt, H., Seppälä, A., Sinnhuber, M., Sukhodolov, T., Stiller, G. P., Tsvetkova, N. D., Verronen, P. T., Versick, S., von Clarmann, T., Walker, K. A., and Yushkov, V.: HEPPA-II model–measurement intercomparison project: EPP indirect effects during the dynamically perturbed NH winter 2008–2009, Atmos. Chem. Phys., 17, 3573–3604, https://doi.org/10.5194/acp-17-3573-2017, 2017.
GATS Inc.: SOFIE NO data, available at: ftp://ftp.gats-inc.com/sofie, last access: 21 January 2019a.
GATS Inc.: SABER O data, available at: http://saber.gats-inc.com/data.php, last access: 21 January 2019b.
Gómez-Ramírez, D., McNabb, J. W. C., Russell III, J. M., Hervig, M. E., Deaver, L. E., Paxton, G., and Bernath, P. F.: Empirical correction of thermal responses in the Solar Occultation for Ice Experiment nitric oxide measurements and initial data validation results, Appl. Optics, 52, 2950–2959, 2013.
Hendrickx, K., Megner, L., Gumbel, J., Siskind, D. E., Orsolini, Y. J., Nesse Tyssøy, H., and Hervig, M. E.: Observation of 27 day cycles in the production and mesospheric descent of EPP-produced NO, J. Geophys. Res., 120, 8978–8988, https://doi.org/10.1002/2015JA021441, 2015.
Hendrickx, K., Megner, L., Marsh, D. R., and Smith-Johnsen, C.: Production and transport mechanisms of NO in the polar upper mesosphere and lower thermosphere in observations and models, Atmos. Chem. Phys., 18, 9075–9089, https://doi.org/10.5194/acp-18-9075-2018, 2018.
Herron, J.: Evaluated chemical kinetics data for reactions of N(2D), N(2P) and N2(A3Σ) in the gas phase, J. Phys. Chem. Ref. Data, 28, 1453, https://doi.org/10.1063/1.556043, 1999.
Jones Jr., M., Emmert, J. T., Drob D. P., and Siskind, D. E.: Middle atmospheric dyanmical sources of the semiannual oscillation in the thermosphere and ionosphere, Geophys. Res. Lett., 44, 12–21, https://doi.org/10.1002/2016GL071741, 2017.
Jones Jr., M., Drob, D. P., Siskind, D. E., McCormack, J. P., Maute, A., McDonald, S. E., and Dymond, K. F.: Evaluating different nudging techniques in the TIME-GCM: The 2010 sudden stratospheric warming period, J. Adv. Model. Earth Sy., https://doi.org/10.1029/2018MS001440, 2018.
Kiviranta, J., Pérot, K., Eriksson, P., and Murtagh, D.: An empirical model of nitric oxide in the upper mesosphere and lower thermosphere based on 12 years of Odin SMR measurements, Atmos. Chem. Phys., 18, 13393–13410, https://doi.org/10.5194/acp-18-13393-2018, 2018.
Knipp, D. J., Pette, D. V., Kilcommons, L. M., Isaacs, T. L., Cruz, A. A., Mlynczak, M. G., Hunt, L. A., and Lin, C. Y.: Thermospheric nitric oxide response to shock-led storms, Space Weather, 15, 325–342, 2017.
Kockarts, G.: Nitric oxide cooling in the terrestrial thermosphere, Geophys. Res. Lett., 7, 137–140, 1980.
Marsh, D. R. and Russell III, J. M.: A tidal explanation for the sunrise/sunset anomaly in HALOE low-latitude nitric oxide observations, Geophys. Res. Lett., 27, 3197–3200, 2000.
Marsh, D. R., Solomon, S. C., and Reynolds, A. E.: Empirical model of nitric oxide, J. Geophys. Res., 109, A07301, https://doi.org/10.1029/2003JA010199, 2004.
Maute, A.: Thermosphere-Ionosphere-Electrodynamics General Circulation Model for the Ionospheric Connection Explorer: TIEGCM-ICON, Space Sci Rev., 212, 523–551, https://doi.org/10.1007/s11214-017-0330-3, 2017.
McCormack, J., Hoppel, K., Kuhl, D, de Wit, R., Stober, G., Espy, P., Baker, N., Brown, P., Fritts, D., Jacobi, C., Mitchell, N., Ruston, B., Swadley, S., Viner, K., Whitcomb, T., and Hibbins, R.: Comparison of mesospheric winds from a high-altitude meteorological analysis system and meteor radar observations during the boreal winters of 2009–2010 and 2012–2013, J. Atmos. Sol.-Terr. Phy., 154, 132–166, https://doi.org/10.1016/j.jastp.2016.12.007, 2017.
Meier, R. R., McLaughlin, B. M., Warren, H. P., and Bishop, J.: Atomic oxygen photoionization rates computed with high resolution cross sections and solar fluxes, Geophys. Res. Lett., 34, L01104, https://doi.org/10.1029/2006GL028484, 2007.
Mlynczak, M., Martin-Torres, F. J., Russell, J., Beaumont, K., Jacobson, S., Kozyra, J., Lopez-Puertas, M., Funke, B., Mertens, C., Gordley, L., Picard, R., Winick, J., Wintersteiner, P., and Paxton, L.: The natural thermostat of nitric oxide emissin at 5.3 µ in the thermosphere observed during the solar storms of April 2002, Geophys. Res. Lett., 30, 2100, https://doi.org/10.1029/2003GL017693, 2003.
Mlynczak, M. G., Hunt, L. A., Mast, J. C., Marshall, B. T., Russell III, J. M., Smith, A. K., Siskind, D. E., Yee, J.-H., Mertens, C. J., Martin-Torres, F. J., Thompson, R. E., Drob, D. P., and Gordley, L. L.: Atomic oxygen in the mesosphere and lower thermosphere from SABER: Algorithm theoretical basis and measurement uncertainty, J. Geophys. Res. 118, 5724–5739, https://doi.org/10.1002/jgrd.50401, 2013.
Mlynczak, M. G., Hunt, L., Russell III, J. M., and Marshall, B. T.: Thermospheric climate indexes: Percentile ranges and adjectival descriptors, J. Atmos Sol.-Terr. Phy., 174, 28–31, https://doi.org/10.1016/j.jastp.2018.04.004, 2018a.
Mlynczak, M. G., Hunt, L. A., Russell III, J. M., and Marshall, B. T.: Updated SABER night atomic oxygen and implications for SABER ozone and atomic hydrogen, Geophys. Res. Lett., 45, 5735–5741 ,https://doi.org/10.1029/2018GL077377, 2018b.
Newnham, D. A., Clilverd, M. A., Rodger, C. J., Hendrickx, K., Megner, L., Kavanagh, A. J., Seppälä, A., Verronen, P. T., Andersson, M. E., Marsh, D. R., Kovács, T., Feng, W., and Plane J. M. C: Observations and modeling of increased NO in the Antarctic polar middle atmosphere, J. Geophys. Res., 123, 6009–6025, https://doi.org/10.1029/2018JA025507, 2018.
Oberheide J. and Forbes, J. M.: Thermospheric nitric oxide variability induced by nonmigrating tides, Geophys. Res. Lett., 35, L16814, https://doi.org/10.1029/2008GL034825, 2008.
Oberheide, J., Mlynczak, M. G., Mosso, C. N., Schroeder, P. M., Funke, B., and Maute, A.: Impact of tropospheric tides on the NO 5.3 µm infrared cooling of the low-latitude thermosphere during solar minimum conditions, J. Geophys. Res., 118, 7283–7293, https://doi.org/10.1002/2013JA019278, 2013.
Pavlov, A. V. and Pavlova, N. M.: Comparisons of electron concentrations in the ionospheric E-layer maximum in spring conditions obtained by calculations and Moscow ionosonde measurements, Geomagn. Aeronomy+, 55, 235–245, https://doi.org/10.1134/S0016793215020140, 2015.
Pedatella, N. M. and Liu, H.-L.: The influence of atmospheric tide and planetary wave variability during sudden stratospheric warmings on the low latitude ionosphere, J. Geophys. Res.-Space, 118, 5333–5347, https://doi.org/10.1002/jgra.50492, 2013.
Randall, C. E., Harvey, V. L., Holt, L. A., Marsh, D. R., Kinnison, D., Funke, B., and Bernath, P. F., Simulation of energetic particle precipitation effects during the 2003–2004 Arctic winter, J. Geophys. Res.-Space, 120, 5035–5048, https://doi.org/10.1002/2015JA021196, 2015.
Roble, R. G. and Ridley, E. C.: A thermosphere-ionosphere-mesosphere-electrodynamics general circulation mdoel (TIME-GCM): Equinox solar cycle minimum simulations (30–500 km), Geophys. Res. Lett., 21, 417–420, https://doi.org/10.1029/93GL03391, 1994.
Russell III, J. M., Gordley, L. L., Park, J. H., Drayson, S. R., Hesketh, W. D., Cicerone, R. J., Tuck, A. F., Frederick, J. E., Harries, J. E., and Crutzen, P. J.: The Halogen Occultation Experiment, J. Geophys. Res., 88, 10777–10797, 1993.
Russell III, J. M., Bailey, S. M., Gordley, L. L., Rusch, D. W., Horanyi, M., Hervig, M. E., Thomas, G. E., Randall, C. E., Siskind, D. E., Stevens, M. H., Summers, M. E., Taylor, M. J., Englert, C. R., Espy, P. J., McClintock, W. E., and Merkel, A. W.: The Aeronomy of Ice in the Mesosphere mission: Overview and early science results, J. Atmos. Sol.-Terr. Phy., 71, 289–299, https://doi.org/10.1016/j.jastp.2008.08.011, 2009.
Sheese, P. E., Strong, K., Gattinger, R. L., Llewellyn, E. J., Urban, J., Boone, C. D., and Smith, A. K.: ODIN observations of Antarctic nighttime NO densities in the mesosphere-lower thermosphere and observations of a lower NO layer, J. Geophys. Res., 118, 7414–7425, https://doi.org/10.1002/jgrd.50563, 2013.
Siskind, D. E., Drob, D. P., Dymond, K. F., and McCormack, J. P.: Simulations of the effects of vertical transport on the thermosphere and ionosphere using two coupled models, J. Geophys. Res., 119, 1172–1185, doi:10.1002/2013JA019116, 2014.
Siskind, D. E., Barth, C. A., and Roble, R. G.: The response of nitric oxide to an auroral storm, 1. Low and mid latitudes, J. Geophys. Res., 94, 16886–16898, 1989a.
Siskind. D. E., Barth, C. A., Evans, D. S., and Roble, R. G.: The response of nitric oxide to an auroral storm, 2. Auroral latitudes, J. Geophys. Res., 94, 16899–16911, 1989b.
Siskind, D. E., Barth, C. A., and Cleary, D. D.: The possible effect of solar soft X-rays on thermospheric nitric oxide J. Geophys. Res., 95, 4311–4317, 1990.
Siskind, D. E., Strickland, D. J., Meier, R. R., Majeed, T., and Eparvier, F. G.: On the relationship between the soft X-ray flux and thermospheric nitric oxide: An update with an improved photoelectron model, J. Geophys. Res., 100, 19687–19694, 1995.
Siskind, D. E., Barth C. A., and Russell III, J. M.: A climatology of nitric oxide in the mesosphere and thermosphere, Adv. Space Res., 21, 1353–1362, 1998.
Siskind D. E., Sassi, F., Randall, C. E., Harvey, V. L., Hervig, M. E., and Bailey, S. M.: Is a high-altitude meteorological analysis necessary to simulate thermosphere-stratosphere coupling?, Geophys. Res. Lett., 42, 8225–8230, https://doi.org/10.1002/2015GL065838, 2015.
Smith-Johnsen, C., Nesse Tyssøy, H., Hendrickx, K., Orsolini, Y., Kumar, G. K., Ødegaard, L.-K. G., Sandanger, M. I., Stordal, F., and Megner, L.: Direct and indirect electron precipitatin effect on nitric oxide in the polar middle atmosphere, using a full-range energy spectrum, J. Geophys. Res.-Space, 122, 8679–8693, https://doi.org/10.1002/2017JA024364, 2017.
Solomon, S. C.: Numerical models of the E-region ionosphere, Adv. Space Res., 37, 1031–1037, 2006.
Solomon, S. C.: The GLobal airglOW Model, GitHub, available at: https://github.com/NCAR/GLOW (last access: 21 January 2019), 2017.
Solomon, S. C. and Qian, L.: Solar extreme-ultraviolet irradiance for general circulation models, J. Geophys. Res., 110, A10306, https://doi.org/10.1029/2005JA011160, 2005.
Solomon, S. C., Bailey, S. M., and Woods, T. N.: Effect of solar soft-rays on the lower ionosphere, Geophys. Res. Lett., 28, 2149–2152, 2001.
Sojka, J. J., Jensen, J., David, M., Schunk, R. W., Woods, T., and Eparvier, F.: Modeling the ionospheric E and F1 regions using SDO-EVE observations as the solar irradiance driver, J. Geophys. Res., 118, 5379–5391, https://doi.org/10.1002/jgra.50480, 2013.
Warren, H.: A solar minimum irradiance spectrum for wavelengths below 1200 Å, Astrophys. J. Suppl. S., 157, 147–173, 2005.
Warren, H., Mariska, J. T., and Wilhelm, K.: High-resolution observations of the solar hydrogen lyman lines in the quiet sun with the SUMER instrument on SOHO, Astrophys. J. Suppl. S., 119, 105–120, 1998.
Yonker, J. D.: Contribution of the first electronically excited state of molecular nitrogen to thermospheric nitric oxide, PhD Thesis, Virginia Tech, Blacksburg, VA, USA, 2013.
Zhang, X., Forbes J., and Hagan, M. E.: The longitudinal variation of tides in the MLT region: 1. Tides driven by tropospheric net radiative heating, J. Geophys. Res, 115, A06316, https://doi.org/10.1029/2009JA014897, 2010a.
Zhang, X., Forbes, J., and Hagan, M. E.: The longitudinal variation of tides in the MLT region: 2. Relative effects of solar radiative and latent heating, J. Geophys. Res, 115, A06317, https://doi.org/10.1029/2009JA014898, 2010b.