the Creative Commons Attribution 4.0 License.
the Creative Commons Attribution 4.0 License.
Effects of solar activity and galactic cosmic ray cycles on the modulation of the annual average temperature at two sites in southern Brazil
Everton Frigo
Francesco Antonelli
Djeniffer S. S. da Silva
Pedro C. M. Lima
Igor I. G. Pacca
José V. Bageston
Quasi-periodic variations in solar activity and galactic cosmic rays (GCRs) on decadal and bidecadal timescales have been suggested as a climate forcing mechanism for many regions on Earth. One of these regions is southern Brazil, where the lowest values during the last century were observed for the total geomagnetic field intensity at the Earth's surface. These low values are due to the passage of the center of the South Atlantic Magnetic Anomaly (SAMA), which crosses the Brazilian territory from east to west following a latitude of ∼ 26∘. In areas with low geomagnetic intensity, such as the SAMA, the incidence of GCRs is increased. Consequently, possible climatic effects related to the GCRs tend to be maximized in this region. In this work, we investigate the relationship between the ∼ 11-year and ∼ 22-year cycles that are related to solar activity and GCRs and the annual average temperature recorded between 1936 and 2014 at two weather stations, both located near a latitude of 26∘ S but at different longitudes. The first of these stations (Torres – TOR) is located in the coastal region, and the other (Iraí – IRA) is located in the interior, around 450 km from the Atlantic Ocean. Sunspot data and the solar modulation potential for cosmic rays were used as proxies for the solar activity and the GCRs, respectively. Our investigation of the influence of decadal and bidecadal cycles in temperature data was carried out using the wavelet transform coherence (WTC) spectrum. The results indicate that periodicities of 11 years may have continuously modulated the climate at TOR via a nonlinear mechanism, while at IRA, the effects of this 11-year modulation period were intermittent. Four temperature maxima, separated by around 20 years, were detected in the same years at both weather stations. These temperature maxima are almost coincident with the maxima of the odd solar cycles. Furthermore, these maxima occur after transitions from even to odd solar cycles, that is, after some years of intense GCR flux. The obtained results offer indirect mathematical evidence that solar activity and GCR variations contributed to climatic changes in southern Brazil during the last century. A comparison of the results obtained for the two weather stations indicates that the SAMA also contributes indirectly to these temperature variations. The contribution of other mechanisms also related to solar activity cannot be excluded.
Keywords. Meteorology and atmospheric dynamics (climatology)
The effects of solar variability on terrestrial climate are discussed in several works (e.g., Wilcox, 1975; Hoyt and Schatten, 1997; Shaviv, 2005; Kirkby, 2007; Solanki et al., 2013). Some of these works suggest that climatic modulation occurs indirectly, through the action of galactic cosmic rays (GCRs) (e.g., Svensmark and Friis-Christensen, 1997). GCRs are predominantly positively electrically charged particles; although these contribute very little to the energy input, they are very important in the ionization processes in the Earth's atmosphere (Carslaw et al., 2002). The entrance of GCRs to the atmosphere is modulated by the magnetic fields of the Sun and the Earth. This solar modulation is due to the time variation in the intensity of the Sun's magnetic field and its polarity reversals (Kudela, 2009), and solar magnetic variations can explain the periodic components of ∼ 11 and ∼ 22 years in GCRs. Geomagnetic modulation occurs in both space and time. The spatial modulation is due to the directional configuration of the geomagnetic field lines, which are vertical close to the magnetic poles and nearly horizontal around the equator, meaning that the GCR flux is at a maximum in the polar regions and at a minimum in equatorial regions. The temporal modulation is due to slow geomagnetic changes, which are usually significant at secular or larger scales. When geomagnetic excursions or polarity reversals occur, the geomagnetic intensity decreases dramatically, causing the GCR flux to increase (Wagner et al., 2000).
Dickinson (1975) indicated that ionization caused by GCR flux variations could influence the mechanisms of cloud formation. Since then, researchers have made much effort to find evidence that could confirm or refute this possible relationship between GCRs and climate. Svensmark and Friis-Christensen (1997) found a positive correlation between GCRs and global cloud cover over an 11-year solar cycle. Svensmark (2007) then complemented the analysis and concluded that the relationship between GCRs and clouds is only valid for low-altitude clouds. The physical mechanism proposed to explain this correlation assumes that an increase in the air ionization in the atmosphere resulting from the increase of GCR flux helps to form aerosols, which may grow and transform into the cloud condensation nuclei needed for water droplet condensation and thus the creation of low-altitude clouds.
Discussions related to possible climatic modulation related to GCRs led to the development of the Cosmics Leaving Outdoor Droplets (CLOUD) experiment. Results obtained from CLOUD have contributed to improvements in the understanding of cloud microphysical phenomena involving nucleation, growth and aerosols. However, the effects of GCRs on clouds remain insufficiently understood (Pierce, 2017). On the other hand, most of the scientific works on this topic are based on an analysis of climatic and paleoclimatic data that aims to evaluate the GCRs–climate relationship, taking into account different scenarios of climate, solar activity and geomagnetic fields at different temporal and spatial scales (e.g., Wagner et al., 2001; Miyahara et al., 2008; Erlykin and Wolfendale, 2011; Svensmark, 2012; Myhre et al., 2014). For example, Kitaba et al. (2017) found a very interesting connection between GCRs and climate at a geological timescale. Through analyzing pollen data from Osaka Bay, Japan, they found that the thermal gradient between land and ocean changed as a consequence of the insolation decrease during geomagnetic polarity reversals. This result is in agreement with the argument made by Svensmark (2007). However, there are few works reporting the possible indirect influence of the geomagnetic field on climatic variations during the last century (e.g., Vieira and da Silva, 2006; Courtillot et al., 2007; Frigo et al., 2013; Campuzano et al., 2016).
Frigo et al. (2013) investigated the spectral coherence between the 11- and 22-year solar activity cycles and the yearly average temperatures recorded during the last century at four weather stations located in southern Brazil's coastal region. This region showed smaller values of geomagnetic intensity in the 20th century, due to the presence of the South Atlantic Magnetic Anomaly (SAMA). The results of wavelet coherence analysis indicated that a periodicity of around 22 years was persistent in climatic data recorded between 1933 and 2008. Moreover, the results indicated a linear relationship between temperature variations and the ∼ 22-year cycle. This result was obtained from data from the two weather stations located nearest to the course of the SAMA. For two stations further away, the 22-year cycle was also persistent during the investigated period, but the statistical results indicated a nonlinear relationship between the bidecadal periodicity and temperature variations. In summary, the results of Frigo et al. (2013) indicated that a climatic modulation of ∼ 22 years, characteristic of GCRs, was persistent during the last century and was possibly at a maximum in the region closest to the center of the SAMA.
The aim of this work is to advance the analyses of Frigo et al. (2013) by investigating the connections between temperature variability, solar activity and GCR variations during the last century, including making use of climatological data recorded in other weather stations in southern Brazil. A time series of the solar modulation potential is used as a proxy for GCR variability.
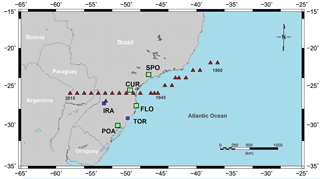
Figure 1Map of southern South America indicating the positions of the center of the SAMA between 1900 and 2015 (red triangles), locations of the weather stations used by Frigo et al. (2013) (green squares) and the weather stations used in this work (blue squares).
The total intensity of the geomagnetic field in southern South America has been strongly affected by the SAMA over the last century. The center of the SAMA, defined as the point at which the lowest geomagnetic intensity value is observed, moved in a southwesterly direction between 1900 and 1945, close to the Brazilian coast (Fig. 1). After 1945, the center of the SAMA crossed southern Brazil, following a straight line in a westerly direction at a latitude of approximately 26∘ S (Hartmann and Pacca, 2009). According to the International Geomagnetic Reference Field (IGRF), the value of the geomagnetic intensity at the center of the SAMA has decreased from about 25 500 nT to about 22 500 nT since 1900. This decrease can amplify certain effects related to the penetration of electrically particles in the Earth's atmosphere.
In the region near to the trajectory of the center of the SAMA, several weather stations have been established. These stations are maintained by the Brazilian National Institute of Meteorology (INMET). Data from São Paulo (SPO), Curitiba (CUR), Florianópolis (FLO) and Porto Alegre (POA) were used in the previous investigation by Frigo et al. (2013). The positions of these stations are indicated in Fig. 1. However, in this study we expand our analysis, using a new, longer temperature time series recorded at the Torres (TOR) and Iraí (IRA) weather stations. TOR is located on the coast, around 260 km south of FLO. IRA is located within the continental area of southern Brazil, around 450 km from the coast. Between 1980 and 1995, the center of the SAMA passed very near to the IRA station.
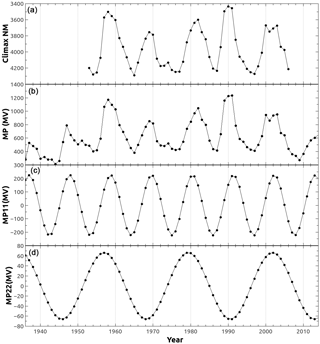
Figure 3(a) Time series of yearly neutron counts at the Climax Observatory, (b) the modulation potential, (c) the approximately 11-year component of the modulation potential, and (d) the approximately 22-year component of the modulation potential. Note that the vertical scale of (a) is inverted to facilitate a comparison with (b).
In order to investigate the possible effects of solar activity on climate in the SAMA region, we use the time series of the yearly mean total sunspot number (SN). SN varies in phase with the solar irradiance and the solar magnetic field intensity, exhibiting a clear cycle of around 11 years. This periodic variation is called the sunspot cycle. Each solar cycle generally receives a number, beginning with the cycle that began in 1755. The SN data, obtained from the website of the Solar Influences Data Analysis Center (http://sidc.oma.be/, last access: 10 January 2018) for the period between 1936 and 2014, are presented in Fig. 2a. Every 11 years, the magnetic polarity of the Sun reverses. Thus, it takes 22 years for the same state of polarity to repeat itself. To investigate possible effects associated with the polarity of the Sun's magnetic field, we multiplied the SN numbers by −1 for the odd solar cycles. This procedure has already been used in previous work (e.g., Souza Echer et al., 2008). The resulting time series, denoted by SN22, is called the double sunspot cycle and is presented in Fig. 2b.
Ground measurements of neutrons, produced by primary cosmic rays when they reach the atmosphere, are the main proxy for GCR variations. However, long-term neutron time series are not available for the beginning of the 20th century. One of the longest (1953–2006) neutron time series was recorded at the Climax Observatory (United States); the data for this were obtained from the Russian Institute of Terrestrial Magnetism, Ionosphere and Radio Wave Propagation website (http://cr0.izmiran.ru/clmx/main.htm, last access: 28 December 2017) and are shown in Fig. 3a. It is well known that GCRs vary in antiphase with the intensity of the Sun's magnetic field over an 11-year solar cycle; due to this, several authors have used sunspot number counts as a proxy for GCR variations. However, more reliable proxies of GCR variability are currently available (e.g., Usoskin et al., 2011; McCracken and Beer, 2015). In this work, we use the proxy proposed by Usoskin et al. (2011), which consists of the reconstruction of the solar modulation potential (MP) for cosmic rays. The MP reconstruction covers the period between 1936 and 2009 and is constructed using data from ground-based ionization chambers (from 1936 to 1951), International Geophysical Year (IGY) neutron monitors (from 1951 to 1964) and a ground-based network of neutron monitors (from 1964 to 2009). The MP reconstruction time series is indicated for studies of cosmic-ray-induced atmospheric ionization, which plays a key role in the GCRs–cloud climate mechanisms. The annual values of MP, extended until 2014, were obtained from the website of the University of Oulu (http://cosmicrays.oulu.fi/phi/Phi_mon.txt, last access: 7 December 2017) and are presented in Fig. 3b. The similarity between the MP, yearly neutron counts at the Climax Observatory (Fig. 3a) and SN time series (Fig. 2a) is clear; this is because the most striking feature of the solar magnetic field in the last century is the approximately 11-year periodicity, which is the main modulation component of GCRs. If the MP varies in phase with solar magnetic field intensity, it varies in antiphase with GCRs.
According to Usoskin et al. (2011) the GCRs, and consequently the MP, show a dominant 11-year cycle and a secondary component of around 22 years. This secondary component is due to the alternation of the Sun's magnetic field polarity and because GCRs are predominantly positively electrically charged particles. During polarity transitions from even to odd solar cycles, the GCR flux remains at a maximum for a period which is longer than that observed during transitions from odd to even cycles (Usoskin et al., 2001). Using a time series iterative regression analysis (ARIST), as described in Rigozo et al. (2005), we can separate and reconstruct the contributions of the individual ∼ 11- and ∼ 22-year components of the MP series. The ARIST method consists of adjusting the observational data using a sinusoidal function, aiming at each iteration to decrease the difference between the adjusted function and the observed data. As a result, ARIST provides the amplitude, the angular frequency and the phase associated with each periodicity detected in the analyzed time series.
For the ∼ 11-year component of MP, referred to here as MP11, values were obtained of 224.171 for the amplitude, 0.09187 for the frequency and 1.87119 for the phase. For the ∼ 22-year component of MP, referred to here as MP22, values were obtained of 66.222 for the amplitude, 0.04460 for the frequency and 6.08605 for the phase. The time series of the ∼ 11- and ∼ 22-year components of MP, computed using the second term of the right-hand side of Eq. (14) in Rigozo (2005), are shown in Fig. 3c and d, respectively. A comparison between MP11 and SN indicates that the two time series are very similar, with maxima and minima almost coincident throughout the investigated period. The maximum time difference observed between the occurrence of maxima or minima in MP11 and SN was 2 years. A comparison between MP22 and SN22 shows that the series are very similar but vary in anti-correlation with each other. The maximum time lag between the occurrence of maxima or minima in MP22 and SN22 is also 2 years. The results of these comparisons indicate that the series of SN and SN22 can be used as proxies for the 11- and 22-year components of GCR variation in studies investigating the effects of GCRs on climate.
The climatological data used in this work consist of the mean annual temperature values calculated for TOR and IRA weather stations (Fig. 4a and b). The annual averages were computed from the recorded monthly averages for the period 1936–2014. The interannual variability and the increasing trend are evident in the TOR and IRA temperature data. The variations in TOR and IRA temperature data are very similar; however, due to the proximity of the Atlantic Ocean, the long-term trend and temperature variation amplitudes for TOR are smaller than those observed for IRA. It is also clear that the occurrence of some maximum temperature events occurred almost simultaneously at the two weather stations. Examples of this are the maxima observed in 1939–1940, 1961, 1977 and 2001–2002. It is interesting to note that these maxima occur next to the SN22 minima, with a few years' time lag. In addition, the average difference between subsequent maxima is around 20 years.
In order to investigate the mathematical relationship between solar activity, GCR and temperature data, wavelet transform coherence (WTC) analysis is used. The WTC is calculated using the individual continuous wavelet transforms and the cross-wavelet transform of the two time series involved. The computational program used was developed by Grinsted et al. (2004) and allows us to estimate values for the coherence coefficient and the phase angles between two series as a function of frequency and time. Coherence values range from 0 to 1, where the highest values are associated with high coherency. Phase angles are represented by arrows, and those that are pointing to the right indicate a linear in-phase relationship. In these cases, the maxima or minima observed in one time series are coincident with the maxima or minima observed in the other. Arrows pointing to the left indicate a linear antiphase relationship. In these cases, a maximum (minimum) observed in one series is coincident with a minimum (maximum) observed in the other series. Arrows pointing in any other direction indicate a nonlinear relationship. In these cases, maxima and minima are observed in the two analyzed time series, but there is a time lag between them. This time lag must be due to the contribution of other variables or phenomena not considered in this analysis. The WTC results also show a cone of influence, which limits a region of statistical confidence for the computed coherence values. In the spectral region outside the cone, edge effects cannot be neglected (Grinsted et al., 2004). This is a limitation of the WTC method and is most important in the analysis of coherence values related to long periodicities.
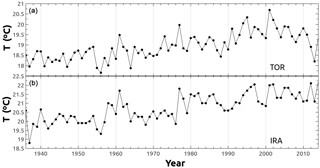
Figure 4Time series of yearly average temperatures at southern Brazil weather stations: (a) TOR and (b) IRA.
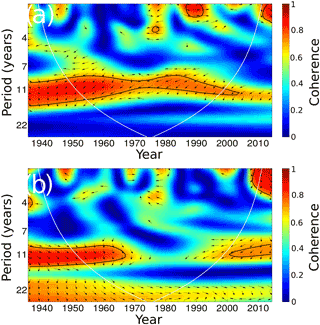
Figure 5Wavelet coherence spectrum between annual average temperature for (a) TOR and SN and (b) IRA and SN. The colors indicate the coherence coefficient: black arrows represent the phase angle between the temperature and the sunspot cycle; black lines limit areas within which coherence values have a confidence level of 95 % against red noise; the white line indicates the area of the cone of influence, below which edge effects cannot be neglected.
The WTC between SN and temperature obtained at TOR is shown in Fig. 5a. The results indicate that the spectral coherence between SN and the temperature at TOR for periodicities of around 11 years was persistent and was higher than 0.65 for the whole period investigated. Phase angles predominantly point downwards. This configuration indicates a nonlinear relationship between the 11-year quasi-cycles and the data from TOR. For IRA, the coherence between SN and temperature was intermittent, as presented in Fig. 5b, and was only higher than 0.65 until 1970 and in the 1995–2014 interval. In these intervals, the phase angles usually point down, suggesting a nonlinear relationship. Coherence values obtained for before ∼ 1950 and after ∼ 2000, shown in Fig. 5a and b, are outside of the cone of influence, and the results should be considered carefully. The same care should be taken in all analyses of the WTC spectra results.
The quasi-11-year cycle in the SN data (see Fig. 2a) is directly correlated to the variations in both solar irradiance and the Sun's magnetic field intensity. Therefore, it is not possible to establish whether the periodicities of ∼ 11 years detected in the temperature time series are due to solar irradiance or to the GCRs. Frigo et al. (2013) found high values for spectral coherence between SN and temperature data for the weather stations of SPO, CUR and FLO. However, these high coherence values were intermittent at these stations during the investigated period. On the other hand, for the POA station, the values of coherence were continuously greater than 0.6. Since the POA station is located about 165 km south of TOR (that is, this weather station is closer to POA than IRA), we would expect the results from TOR to be more similar to POA and the reverse to be true for IRA.
Figure 6a shows the WTC between SN22 and the TOR temperature data. The WTC showed high values of coherence near 22-year periodicity between 1975 and 2014. For this time interval, the phase angle analysis arrows predominantly point left. This configuration indicates a linear antiphase relationship between the time series. In Fig. 5b, it can be seen that the WTC between SN22 and the temperature at IRA was continuous and showed high coherence values for periodicities around 22 years. For these periodicities, the phase angles point mainly to the left, indicating a linear antiphase relationship between SN22 and temperature during the 1936–2014 time interval. These results are in agreement with the results of Frigo et al. (2013), which indicate a linear relationship between SN22 and temperature for the weather stations closest to the trajectory of the center of the SAMA.
The time series of SN22 is an index related to the Sun's magnetic polarity, which influences the GCR variability. Therefore, high values of spectral coherence between SN22 and temperature for periodicities of around 22 years must be associated with GCR variations. Frigo et al. (2013) found high and continuous values of coherence for the four weather stations investigated. However, the mathematical evidence for a linear relationship between SN22 and temperature was only obtained for the SPO, CUR and FLO stations. These three stations are the closest to the path followed by the center of the SAMA over southern Brazil in the last century. A similar situation is observed when the results for TOR and IRA are compared. A linear relationship between SN22 and temperature is observed for the IRA weather station, closest to the path of the SAMA. This result reinforces the indirect influence of the geomagnetic field (through the SAMA) and the solar activity (possibly through GCRs) on climate in southern Brazil.
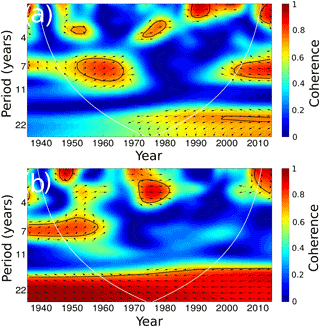
Figure 6Wavelet coherence spectrum between annual average temperature for (a) TOR and SN22 and (b) for IRA and SN22. The black arrows represent the phase angle between the temperature and the double sunspot cycle. Further legend information is the same as for Fig. 5.
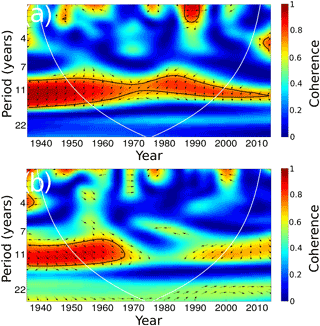
Figure 7Wavelet coherence spectrum between annual average of the modulation potential and temperature for (a) TOR and (b) IRA. The black arrows represent the phase angle between the modulation potential and the temperature data. Further legend information is the same as for Fig. 5.
The wavelet coherence spectra between MP and the temperature data from TOR and IRA are shown in Fig. 7a and b. As suggested in the comparison between Figs. 2a and 3b, and considering periodicities of around 11 years, the results of the coherence between MP and temperature are very similar to the results for the coherence between SN and temperature. For periodicities of around 22 years, no significant values of coherence are observed in the TOR data. Low coherence values of around 0.4 were obtained for the IRA data for the entire period investigated. These results, unlike those observed for the WTC between SN22 and temperature data, do not indicate a continuous relationship between GCRs and climate for bidecadal periodicities. This difference can be explained by the fact that the ∼ 22-year cycle is not a dominant periodicity in the GCR spectrum, meaning that the coherence values for this periodicity in the WTC spectrum are small.
In addition, since the maxima temperature peaks are evident and the solar magnetic cycle modulation of the GCR variations is known, it may be that the influence of the bidecadal cycle only manifested as a ∼ 22 year peak in temperature data rather than a continuous modulation following a periodic function during the last century. As the ∼ 11-year periodicity modulation related to the solar irradiation or GCRs is present in the temperature data, we suggest that following transitions from even to odd solar cycles, some physical mechanism connecting solar activity, GCRs and climate is strengthened, causing the observed bidecadal maxima in yearly averaged temperature data for southern Brazil. Moreover, the differences in the results obtained for TOR and IRA may be because TOR is located in the coastal region, while IRA is located in the continental region. Krahenbuhl (2015) showed that the correlation between solar activity and GCR variation with climatic variation is dependent on the geographical position. The differences in correlations are significant when the marine and continental positions are compared.
Another possible mechanism that can explain the relationship between the solar activity and the temperature variations in southern Brazil involves the high-energy particle precipitation (EPP). Occurrences of EPP in the SAMA region coming from the Van Allen radiation belts are discussed in many works (e.g., Martin et al., 1972; Trivedi et al., 2005). Based on low-altitude satellite measurements, Grigoryan et al. (2008) find that the point of maximum flux of electrons, protons, neutrons and gamma radiation shows positions and drifts similar to that observed for the center of the SAMA in recent decades. Experimental results suggest that the precipitation of relativistic electrons from the radiation belt can influence the reactive nitrogen budget in the higher portions of the atmosphere, but the physical mechanism linking this influence with the climate of the lower atmosphere has not yet been properly established (Mironova et al., 2015).
In addition to the mechanisms already mentioned, two other possible mechanisms involving variations in the solar irradiance have been suggested to explain the relationships between solar activity and climate. These are described in detail in Solanki et al. (2013). According to the first of these mechanisms, radiation at the visible wavelength absorbed by the Earth's surface initiates a mechanism that develops from the bottom to the top, which influences the atmosphere and the oceans through changes in the intertropical convergence zone and tropical circulations. The second mechanism, which develops from the top to the bottom, is related to variations in the intensity of UV radiation in the stratosphere. This mechanism is based on the fact that the variations in solar activity during an 11-year cycle are more intense at shorter wavelengths, which include UV radiation. The variations in UV radiation modify the concentrations of ozone and lead to changes in the atmospheric circulation dynamics. Solanki et al. (2013) also suggest that these two mechanisms can work together.
This work aimed to investigate climatic modulation by solar activity and GCRs in long-term temperature series recorded at two weather stations located in southern Brazil. The results indicated that periodicities of ∼ 11 and ∼ 22 years may have modulated the climatic variability in southern Brazil between 1936 and 2014. Four temperature maxima were observed in IRA and TOR weather station records. The results of WTC analysis indicate a linear relationship between SN22 and temperature for the IRA station; this result is indirect mathematical evidence in favor of the maximization of the GCR effects on climate at IRA, due to its proximity to the trajectory of the center of the SAMA. The linear antiphase relationship between SN22 and the temperature observed at IRA indicates that the minima of SN22 are related to the maxima in temperature. The minima of SN22 correspond to the maxima of odd solar cycles, which in turn correspond to minima in the GCR flux variations. Thus, the results obtained for IRA corroborate the mechanism of Svensmark (2007), which suggests that temperature maxima (minima) are related to GCR minima (maxima).
Although the maxima of SN22 are associated with the maxima of even solar cycles, and should be associated with temperature maxima peaks, the temperature data do not show significant temperature maxima corresponding to the SN22 maxima. The more striking temperature maxima observed occur after transitions from even to odd solar cycles. During these transitions, the maximum in the GCR flux remains over a longer time than that observed in transitions from odd to even solar cycles. This may be a feature of the modulation of temperature by solar activity and GCRs in southern Brazil, considering the values of the geomagnetic field and solar activity observed during the 1936–2014 period. Other mechanisms also associated with solar activity, such as EEP, solar irradiance and UV radiation, cannot be discarded as factors contributing to the modulation of the southern Brazil temperature variations. Since all of these mechanisms are related to solar activity, it is possible that they act together. From the results obtained in this work, it is not possible to quantify the relative contribution of each of these mechanisms to the investigated temperature data.
The results obtained in this work constitute indirect mathematical evidence that solar activity and GCR cycles may contribute to the modulation of the climate in the South Atlantic Magnetic Anomaly region. Future work is expected to include the investigation of data from the last century in terms of other climatic variables, such as rainfall and cloudiness, using data from other weather stations in southern Brazil and satellite data from the upper levels of the Earth's atmosphere (mesosphere–lower thermosphere).
Temperature data recorded in the stations of IRA and TOR were provided by the Brazilian National Institute of Meteorology (Instituto Nacional de Meteorologia – INMET). These data can be accessed in the Meteorological Database for Teaching and Research (Banco de Dados Meteorológicos para Ensino e Pesquisa – BDMEP) of the INMET, on the website http://www.inmet.gov.br/portal/index.php?r=bdmep/bdmep (INMET, 2018). Pre-1960 data are being digitized and should be available online soon. These ancient data, not yet digitized, can be obtained from the INMET units.
The authors declare that they have no conflict of interest.
This article is part of the special issue “Space weather connections to near-Earth space and the atmosphere”. It is a result of the 6o Simpósio Brasileiro de Geofísica Espacial e Aeronomia (SBGEA), Jataí, Brazil, 26–30 September 2016.
The authors thank the Brazilian National Institute of Meteorology for
providing the temperature data for the Iraí and Torres weather
stations. The authors also thank the Universidade Federal do Pampa
(Unipampa), the Instituto de Astronomia, Geofísica e Ciências
Atmosféricas da Universidade de São Paulo (IAG/USP) and the Southern
Regional Center for Space Research (CRS) of the National Institute for Space
Research (INPE) for institutional support. Everton Frigo is grateful to the
Conselho Nacional de Desenvolvimento Científico e Tecnológico
(CNPq) for financial support (universal call 01/2016, process
429068/2016-6). The authors also thank the reviewers for their important
contributions to this work.
The topical editor, Alisson Dal Lago, thanks three anonymous referees for help in evaluating this paper.
Campuzano, S. A., De Santis, A., Pavón-Carrasco, F. J., Osete, M. L., and Qamili, E.: Transfer Entropy between South Atlantic Anomaly and Global Sea Level for the last 300 years, Nat. Hazards Earth Syst. Sci. Discuss., https://doi.org/10.5194/nhess-2016-56, in review, 2016.
Carslaw, K. S., Harrison, R. G., and Kirkby, J.: Cosmic rays, clouds, and climate, Science, 298, 1732–1736, 2002.
Courtillot, V., Gallet, Y., Le Mouël, J.-L., Fluteau, F., and Genevey, A.: Are there connections between the Earth's magnetic field and climate?, Earth Planet. Sc. Lett., 253, 328–339, 2007.
Dickinson, R. E.: Solar variability and the lower atmosphere, B. Am. Meteorol. Soc., 56, 1240–1248, 1975.
Erlykin, A. D. and Wolfendale, A. W.: Cosmic ray effects on cloud cover and their relevance to climate change, J. Atmos. Sol.-Terr. Phy., 73, 1681–1686, 2011.
Frigo, E., Pacca, I. G., Pereira-Filho, A. J., Rampelloto, P. H., and Rigozo, N. R.: Evidence for cosmic ray modulation in temperature records from the South Atlantic Magnetic Anomaly region, Ann. Geophys., 31, 1833–1841, https://doi.org/10.5194/angeo-31-1833-2013, 2013.
Grigorian, O. R., Romashova, V. V., and Petrov, A. N.: SAA drift: Experimental Results, Adv. Space Res., 41, 76–78, 2008.
Grinsted, A., Moore, J. C., and Jevrejeva, S.: Application of the cross wavelet transform and wavelet coherence to geophysical time series, Nonlin. Processes Geophys., 11, 561–566, https://doi.org/10.5194/npg-11-561-2004, 2004.
Hartmann, G. A. and Pacca, I. G.: Time evolution of the South Atlantic Magnetic Anomaly, Ann. Brazilian Acad, Sciences, 81, 243–255, 2009.
Hoyt, D. V. and Schatten, K. H.: The role of the sun in climate change, Oxford University Press, New York, USA, 1997.
INMET: Temperature data recorded in the stations of IRA and TOR, available at: http://www.inmet.gov.br/portal/index.php?r=bdmep/bdmep, last access: 27 March 2018.
Kirkby, J.: Cosmic Rays and Climate, Surv. Geophys., 28, 333–375, 2007.
Kitaba, I., Nakagawa, T., Hyodo, M., Katoh, S., Dettman, D. L., and Sato, H.: Geological support for the Umbrella Effect as a link between geomagnetic field and climate, Sci. Rep., 7, 40682, https://doi.org/10.1038/srep40682, 2017.
Krahenbuhl, D. S.: Investigating a solar influence on cloud cover using the North American Regional Reanalysis data, J. Space Weather Spac., 5, 1–10, https://doi.org/10.1051/swsc/2015012, 2015.
Kudela, K.: On energetic particles in space, Acta Phys. Slovaca, 59, 537–652, 2009.
Martin, I. M., Rai, D. B., da Costa, J. M., Palmeira, R., and Trivedi, N. B.: Enhanced Electron Precipitation in Brazilian Magnetic Anomaly in Association with Sudden Commencement, Nature Physical Science, 240, 84–86, 1972.
McCracken, K. G. and Beer, J.: The Annual Cosmic-Radiation Intensities 1391–2014; The Annual Heliospheric Magnetic Field Strengths 1391–1983, and Identification of Solar Cosmic-Ray Events in the Cosmogenic Record 1800–1983, Sol. Phys., 290, 3051–3069, 2015.
Mironova, I. A., Aplin, K. L., Arnold, F., Bazilevskaya, G. A., Harrison, R. G., Krivolutsky, A. A., Nicoll, K. A., Rozanov, E., Turunen, E., and Usoskin, I. G.: Energetic Particle Influence on the Earth's Atmosphere, Space Sci. Rev., 194, 1–96, https://doi.org/10.1007/s11214-015-0185-4, 2015.
Miyahara, H., Yokoyama, Y., and Masuda, K.: Possible link between multi-decadal climate cycles and periodic reversals of solar magnetic field polarity, Earth Planet. Sc. Lett., 272, 290–295, 2008.
Myhre, G., Shindell, D., Bréon, F.-M., Collins, W., Fuglestvedt, J., Huang, J., Koch, D., Lamarque, J.-F., Lee, D., Mendoza, B., Nakajima, T., Robock, A., Stephens, G., Takemura, T., and Zhang, H.: Anthropogenic and Natural Radiative Forcing, in: Climate Change 2013: The Physical Science Basis. Contribution of Working Group I to the Fifth Assessment Report of the Intergovernmental Panel on Climate Change, edited by: Stocker, T. F., Qin, D., Plattner, G.-K., Tignor, M. S., Allen, K., Boschung, J., Nauels, A., Xia, Y., Bex V., and Midgley, P. M., Cambridge University Press, Cambridge, United Kingdom and New York, NY, USA, 659–740, ISBN: 978-1-107-66182-0, 2014.
Pierce, J. R.: Cosmic rays, aerosols, clouds, and climate: Recent findings from the CLOUD experiment, J. Geophys. Res.-Atmos., 122, 8051–8055, https://doi.org/10.1002/2017JD027475, 2017.
Rigozo, N. R., Nordemann, D. J. R., Echer, E., Vieira, L. E. A., and Faria, H. H.: Comparative study between four classical spectral analysis methods, Appl. Math. Comput., 168, 411–430, 2005.
Shaviv, N. J.: On climate response to changes in the cosmic ray flux and radiative budget, Geophys. Res., 110, A08105, https://doi.org/10.1029/2004JA010866, 2005.
Solanki, S. K., Krivova, N. A., and Haigh, J. D.: Solar Irradiance Variability and Climate, Annu. Rev. Astron. Astr., 51, 311–351, https://doi.org/10.1146/annurev-astro-082812-141007, 2013.
Souza Echer, M. P., Echer, E., Nordemann, D. J. R., Rigozo, N. R., and Prestes, A.: Wavelet analysis of a centennial (1895- 1994) southern Brazil rainfall series (Pelotas, 31∘46′19′′ S 52∘20′33′′ W), Clim. Change, 87, 489–497, 2008.
Svensmark, H.: Cosmoclimatology: a new theory emerges, News Rev. Astron. Geophys., 48, 1.18–1.24, 2007.
Svensmark, H.: Evidence of nearby supernovae affecting life on Earth, Mon. Not. R. Astron. Soc., 423, 1234–1253, 2012.
Svensmark, H. and Friis-Christensen, E.: Variation of Cosmic Ray Flux and Global Cloud Coverage – a Missing Link in Solar Climate Relationships, J. Atmos. Sol.-Terr. Phy., 59, 1225–1232, 1997.
Trivedi, N. B., Abdu, M. A., Pathan, B. M., Dutra, S. L. G., Schuch, N. J., Santos, J. C., and Barreto, L. M.: Amplitude enhancement of SC(H) events in the South Atlantic anomaly region, J. Atmos. Sol.-Terr. Phy., 67, 1751–1760, 2005.
Usoskin, I., Mursula, K., Kananen, H., and Kovaltsov, G. A.: Dependence of cosmic rays on solar activity for odd and even solar cycles, Adv. Space Res., 27, 571–576, 2001.
Usoskin, I. G., Bazilevskaya, G. A., and Kovaltsov, G. A.: Solar modulation parameter for cosmic rays since 1936 reconstructed from ground-based neutron monitors and ionization chambers, J. Geophys. Res., 116, A02104, https://doi.org/10.1029/2010JA016105, 2011.
Vieira, L. E. A. and da Silva, L. A.: Geomagnetic modulation of clouds effects in the Southern Hemisphere Magnetic Anomaly through lower atmosphere cosmic ray effects, Geophys. Res. Lett., 33, L14802, https://doi.org/10.1029/2006GL026389, 2006.
Wagner, G., Masarik, J., Beer, J., Baumgartner, S., Imboden, D., Kubik, P. W., Synal, H.-A., and Suter, M.: Reconstruction of the geomagnetic field between 20 and 60 kyr BP from cosmogenic radionuclides in the GRIP ice core, Nucl. Instrum. Meth. B, 172, 597–604, 2000.
Wagner, G., Livingstone, D. M., Masarik, J., Muscheler, R., and Beer, J.: Some results relevant to the discussion of a possible link between cosmic rays and the Earth's climate, J. Geophys. Res., 106, 3381–3387, 2001.
Wilcox, J. M.: Solar activity and the weather, J. Atmos. Sol.-Terr. Phy., 37, 237–256, 1975.